A guide to understanding and analyzing factor attribution on Boosted Insights.
Table of Content
- What is factor attribution?
- What are style factors?
- Factor Attribution Chart
- Approaches for calculating factor attribution
What is factor attribution?
Factor attribution is the methodology of using a stock or strategy’s factor exposure to explain its risk and reward. Understanding the impact of various factors on your stock or strategy can help you reduce risk and improve returns through several approaches that combat unwanted exposures within Boosted Insight.
What are style factors?
Style factors are logical grouping of the underlying data that drive long-term risks and returns. Boosted Insights identifies nine primary style factors, but any number of style factors can be defined and each style factor can be defined in many ways.
For example, Size typically includes data such as the Market Value of Equity but can sometimes have Total Assets and Total Sales. As a style factor, it measures the risks and returns between large and small companies.
Most of our primary style factors are common in finance, but Market and Idiosyncratic factors are unique to Boosted Insights.
- Market - Market factor includes data representing broad market risks used to explain risks and returns for a particular stock or strategy.
- Idiosyncratic - Idiosyncratic factor includes data representing stock-specific risks used to explain risks and returns for a particular stock or strategy.
Factor Attribution Chart
You can use the Factor Attribution Chart to see how primary style factors contribute to your strategy’s Return and Beta.
To access the chart, simply click on your strategy and select Factor Attribution from the Graphed Metric section.
Hovering over a date, you can see more details on return and beta attribution.
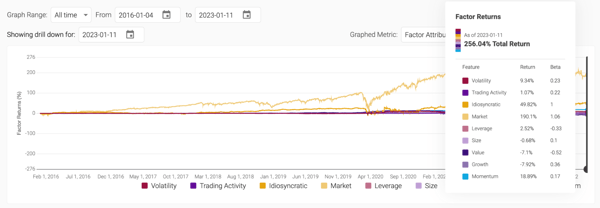
You can click on a specific factor to disable it from the chart.
Approaches for calculating factor attribution
Existing approaches for factor attribution
Time-series Regression (ie. Fama-French Model) - Time-series regressions look at the relationship between a stock’s factor exposures and returns over time to determine factor attribution. This approach works well for factors that are slow to change but poorly for factors that vary more with time because factor returns are calculated before they are realized (ex-ante). More stable factors mean better factor return predictions.
Cross-sectional Regression (ie. Barra Model) - Cross-sectional regressions look at the relationship between factor exposures and returns across multiple stocks at a single point in time to determine factor attribution. This approach calculates factor returns after they are realized (ex-post), which allows for factor attribution for factors that vary more with time. However, the drawdown is over-attributing returns to a particular factor when multiple factors contribute to returns.
Y = 𝛼 + β1F1 + β2F2 … βnFn + ε
Boosted.ai approach for factor attribution
Boosted.ai Regression Methodology - Boosted.ai’s regression keeps factor returns and factor exposures interpretable by relying on regressions only to scale exposures and returns appropriately with each other using a scaling factor (δ). This approach avoids holding time-varying measurements constant and over-ascribing collinear returns to factors.
Y = 𝛼 + β1δ1F1 + β2δ2F2 … βnδnFn + ε
To further improve interpretability and reduce overfitting returns to a factor, we take a stock-up approach. Performing attribution at the stock level and then cumulating the factors up to the portfolio level allows us to track 100% of the attribution back to each stock.
In summary, Boosted.ai provides a more accurate and intuitive understanding of how key factors contribute to a stock or strategy's risks and returns. To see how factors are affecting your results, log into Boosted Insights and see Factor Attributions.